Weijian Luo
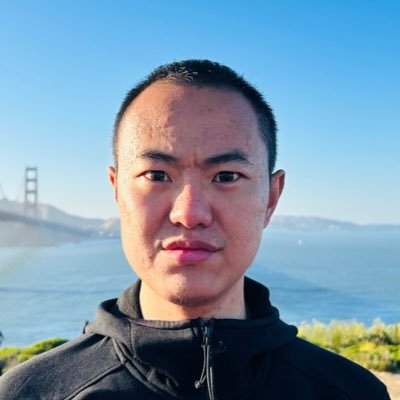
Weijian is RedStar Senior research scientist of Humane Intelligence (hi) lab of Xiaohongshu (RedNote) Inc, Beijing. He obtained his Doctoral Degree in Statistics and Generative Modeling from the School of Mathematical Sciences, Peking University. He received his M.S. Degree in Applied Statistics from the School of Mathematical Sciences also from Peking University, and his B.S. degree in Mathematics from University of Science and Technology of China (USTC).
Research Interests: Weijian's early work had set the theory and practices for modern one-step text-to-image generative models. Currently, Weijian leads the research direction of large generative understanding models in hi-lab. His team focuses on developing cutting-edge, efficient generative understanding models that can reason, understand humane intentions, and generate vision-audio responses in a real-time manner. Weijian also leads the research direction of next-generation generative models, including one-step text-to-image and video models at scale.
Call for Talents: Weijian's team in Beijing is actively hiring talented research scientists and engineers. The team encourages candidates with strong track records and unparalleled curiosity about next-gen generative understanding models to apply for the RedStar Research Scientist program, as well as the ACE intern program.
Academic Services: Weijian is invited as a reviewer for academic journals including Nature Communications (NC), Journal of Machine Learning Research (JMLR), IEEE Transactions on Image Processing (TIP), IEEE Transactions on Neural Networks and Learning Systems (TNNLS), and Pattern Recognition (PR). He also reviews for top AI Conferences including NeurIPS, ICML, ICLR, CVPR, ICCV, AISTATS, UAI, ACM-MM, etc;
Contact: pkulwj1994 at icloud dot com
Selected Talks:
- Google Deepmind Research invited me to deliver a talk in 12th Nov, 2024 on one-step cross-modality generative models. Please check out the slides through A Path to Human-preferred One-step Text-to-image Generative Models.
- Research Talk @ Genmo AI, Online, 3rd Jan, 2025: RLHF for Text-to-image Models and Beyond.
- Invited Talk @ Biomedical Engineering lab, Peking University, 25th Oct, 2024: Recent Progress on Diffusion Distillations.
- Invited Talk @ MAPLE lab, Westlake University, 20th Oct, 2024: Efficient Generative Models.
News:
- 16th June, 2025: one preprint paper is public on Arxiv.
Dive3D: Diverse Distillation-based Text-to-3D Generation via Score Implicit Matching (Bai et al., 2025) Dive3D introduces Score-implicit Matching techniques to text-to-3D generation, which significantly improves generative diversity as well as quality. - 25th May, 2025: one preprint paper is public on Arxiv.
Uni-Instruct: One-step Diffusion Model through Unified Diffusion Divergence Instruction (Wang et al., 2025) Uni-Instruct unifies over 10 existing one-step diffusion distillation in theory, with an absolute SoTA one-step FID of 1.02 on ImageNet64 generation benchmark. - 4th May, 2025: one paper accepted by International Conference of Machine Learning (ICML) 2025.
Diff-Instruct*: Towards Human-Preferred One-step Text-to-image Generative Models (Luo et al., 2024) We introduced a novel score-based PPO algorithm for RL fine-tuning of 1-step text-to-image generative models. Our open-sourced 0.6B DIstar-SDXL-1step model outperforms the 12B FLUX-dev diffusion model in human preference scores. - 19th March 2025: one preprint paper is public on Arxiv.
Rewards Are Enough for Fast Photo-Realistic Text-to-image Generation (Luo et al., 2025).
We present a novel finding: reward maximization with proper regularizations can effectively train large-scale few-step text-to-image generative models. - 27th February 2025: one paper accepted by CVPR 2025.
Schedule On the Fly: Diffusion Time Prediction for Faster and Better Image Generation (Ye et al., 2024).
We explore a novel attempt to use reinforcement learning for training diffusion models, with a very strong diffusion model with adaptive generation steps. - 23th January 2025: one paper accepted by ICLR 2025.
Consistency Models Made Easy.
We introduce a set of practical techniques for efficient training of consistency models, together with a comprehensive study on the Scaling Law of consistency models. - 5th Dec 2024: One pre-print is public on Arxiv.
Self-Guidance: Boosting Flow and Diffusion Generation on Their Own (Li et al., 2024).
Self-guidance can improve human hands and bodies of images generated by diffusion or flow models. - 1st Dec 2024: One pre-print is public on Arxiv.
Schedule On the Fly: Diffusion Time Prediction for Faster and Better Image Generation (Ye et al., 2024).
We introduced an approach for training variable-time-schedule diffusion models using reinforcement learning. - 21st Nov 2024: One single-author paper accepted by Transactions on Machine Learning Research (TMLR).
Diff-Instruct++: Training One-step Text-to-image Generator Model to Align with Human Preferences (Luo, 2024).
Diff-Instruct++ is the first work on preference alignment of one-step text-to-image generative models, opening the preference alignment with the distillation of diffusion and flow models. - 12th Nov 2024: Delivered an invited talk at the Google Deepmind Diffusion Reading Group titiled A Path to Human-preferred One-step Text-to-image Generative Models. Check the [Slides] here.
- 30th Oct 2024: Be invited to give an (internal) online academic talk in the Google Deepmind research team on 12th Nov. The talk title is One-step Text-to-image Generative Models: from Diffusion Distillation to Human-preference Alignment. In this talk, I will share some exciting progress in improving human preferences for one-step and few-step text-to-image generative models through the lens of Reinforcement Learning using Human Feedback (RLHF). Readers can refer to Diff-Insruct++ and Diff-Insruct* for technical details.
- 25th Oct 2024: An invited talk delivered at the Biomedical Engineering lab led by Dr. Sun at Peking University, Beijing, China. The talk is on Recent Progresses on Diffusion Distillation.
- 20th Oct 2024: Had an academic visit to MAPLE lab led by Dr. Qi in Westlake University, Hangzhou, China. Delivered a talk on Efficient Generative Models to lab members.
- 18th Oct 2024: one reprint released on Arxiv.
One-step Flow Matching Generators (Huang et al., 2024).
We introduce a novel method to distill the flow-matching-based Stable Diffusion 3 model into strong one-step generators. - 18th Oct 2024: one reprint released on Arxiv.
Diff-Instruct*: Towards Human-Preferred One-step Text-to-image Generative Models (Luo et al., 2024).
This paper introduces the Diff-Instruct*, a novel approach to train human-preferred large-scale one-step text-to-image generative models through the lens of online RLHF with general score-based constraints. The resulting one-step 0.6B DiT-DI* model achieves a SoTA HPSv2.0 score of 28.70. - 17th Oct 2024: one reprint released on Arxiv.
Diff-Instruct++: Training One-step Text-to-image Generator Model to Align with Human Preferences (Luo, 2024).
This paper introduces the Diff-Instruct++, the first attempt at human preference alignment of large-scale one-step text-to-image generative models. The aligned one-step 0.6B DiT-DI++ model achieves a leading HPSv2.0 score of 28.48. - 14th Oct 2024:I defended my PhD Thesis in 14th Oct in Peking University. I feel humbled and grateful to be loved and helped by great advisors, family, and awesome friends.
- 26th Sep 2024:one paper accepted by NeurIPS 2024.
One-step Diffusion Distillation Through Score Implicit Matching (Luo et al., NeurIPS 2024).
We introduce the score implicit matching, a novel one-step diffusion distillation approach with an amazing one-step text-to-image generative model. Appreciation to Prof. Zico Kolter and Prof. Guojun Qi. - 20th Jun 2024: one preprint released on Arxiv.
Consistency Models Made Easy (Geng et al., 2024).
We introduce a set of practical techniques for efficient training of consistency models, together with a comprehensive study on the Scaling Law of consistency models. - 24th Apr 2024:one paper accepted by ICML 2024.
Variational Schrödinger Diffusion Models (Deng et al., ICML 2024).
We introduce an efficient simulation-free Schrödinger diffusion model, with wide applications for image and time-series generation. Congratulations to Yixin and Dr. Deng. - 26th Sep 2023:oone paper accepted by NeurIPS 2023.
Diff-instruct: A Universal Approach for Transferring Knowledge from Pre-trained Diffusion Models (Luo et al., NeurIPS 2023).
Diff-Instruct is a one-step diffusion distillation approach through the lens of distribution matching, with applications on text-to-3D generation and improving GAN generators. - 26th Sep 2023:one paper accepted by NeurIPS 2023.
Entropy-based Training Methods for Scalable Neural Implicit Samplers (Luo et al., NeurIPS 2023).
We introduced two interesting training approaches for neural implicit samplers termed KL and Fisher training. - 26th Sep 2023:one paper accepted by NeurIPS 2023.
SA-Solver: Stochastic Adams Solver for Fast Sampling of Diffusion Models (Xue et al., NeurIPS 2023).
We introduced a novel diffusion sampler based on the Stochastic Adam theory, integrated for PixelArt-alpha diffusion models. - 26th Sep 2023:one paper accepted by NeurIPS 2023.
Enhancing Adversarial Robustness via Score-based Optimization (Zhang et al., NeurIPS 2023).
We introduced a novel optimization-based adversarial defense based on pre-trained diffusion models. - 9th Apr 2023: one paper released on Arxiv.
A Comprehensive Survey on Knowledge Distillation of Diffusion Models (Luo, 2023).
The first survey on diffusion distillation and knowledge transferring of diffusion models.
Friends with whom I have worked on projects:
- J. Zico Kolter, Professor, Director of the Machine Learning Department, Carnegie Mellon University (CMU).
- Guo-jun Qi, Professor, IEEE Fellow, Director of MAPLE Lab of Westlake University.
- Kenji Kawaguchi, Presidential Young Professor at Department of Computer Science National University of Singapore.
- He Sun, PhD, tenure-track Assistant Professor at Peking University.
- Tianyang Hu, PhD, Incoming Assistant Professor at the Chinese University of Hong Kong, Shenzhen (CUHK-Shenzhen).
- Wenzheng Chen, PhD, tenure-track Assistant Professor at Peking University.
- Wei Deng, PhD, Research Scientist at Morgan Stanley, New York.
- Ricky Tian Qi Chen, PhD, Research Scientist at Meta Fundamental AI Research (FAIR), New York.
- Seth Forsgren, BS from Princeton, CEO and the founder of Riffusion AI, San Francisco.
- Hayk Martiros, MS from Stanford, CTO and the co-founder of Riffusion AI, technical VP of Skydio.
- Debing Zhang, PhD, Director of Artificial General Intelligence (AGI) team of RedNote, aka Xiaohongshu Inc.
Previous students whom I have advised or worked with:
- Weimin Bai, PhD student at Peking University, co-advised with Professor He Sun.
- Yubo Li, Undergraduate. student at Tsinghua University, and incoming PhD student at Peking University, co-advised by Professor He Sun.
- Yifei Wang, Undergraduate student at Peking University, and incoming PhD student at Rice University (Houston). Co-advised with Professor He Sun.
- Le Zhuo, Incoming CS PhD student of MMLab at the Chinese University of Hong Kong.
- Zemin Huang, CS PhD student of the joint PhD Program of Zhejiang University and Westlake University, co-advised with Professor Guo-jun Qi.
- Tiancheng Li, CS PhD student of the joint PhD Program of Zhejiang University and Westlake University, co-advised with Professor Guo-jun Qi.
- Zilyu Ye, CS undergraduate student of South China University of Technology, co-advised with Professor Guo-jun Qi.
- Yuxuan Gu, Incoming M.S. student at Peking University, co-advised with Professor He Sun.
- Chaowei Liu, National University of Singapore (NUS), co-advised with Professor Guo-jun Qi.